Psychometric modeling and the evaluation of rater effects in performance assessments
SEHD News, 03-25-2021
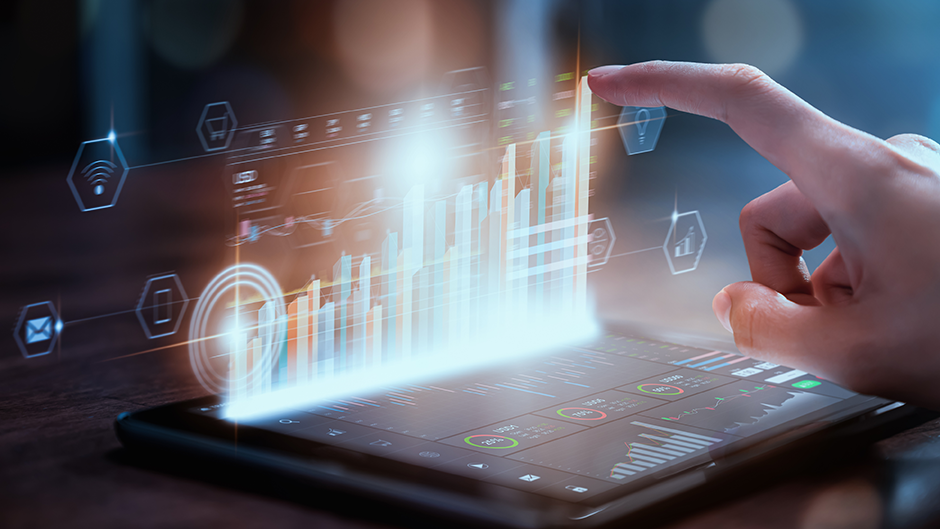
Jue Wang, Ph.D. is an assistant professor in the Research, Measurement & Evaluation Program under the Department of Educational and Psychological studies. Her research emphasizes three inter-related areas: (a) advancing Rasch measurement theory and multilevel item response models for solving measurement problems, (b) development of unfolding techniques for evaluating rater-mediated assessment systems, and (c) exploring rater effects and perceptions in operational scoring activities. She has published in leading journals related to measurement such as Educational and Psychological Measurement, Journal of Educational Measurement, Educational Measurement: Issues and Practices, Assessing Writing, and Measurement: Interdisciplinary Research and Perspectives. She has recently published a book with Professor George Engelhard entitled Rasch models for solving measurement problems: Invariant measurement in the social sciences by Sage as a part of their Quantitative Applications in the Social Sciences (QASS) series.
Her recent research demonstrated the effectiveness of using unfolding models to examine rater effects in performance assessments. Performance assessments are crucially important for examining students’ placement, promoting teaching and learning, and increasing future successes. These assessments require human raters to judge student performances. Enhancing rating quality would make the system more valid, reliable, and fair in education and throughout society. Jue’s work has revealed the great potential of unfolding models in evaluating raters’ decision-making processes. She is currently developing a computerized adaptive testing (CAT) procedure that utilizes an unfolding model for evaluating rater scoring proficiency. This project will further facilitate the creation of a self-paced and computer-based adaptive rater training program. Based on CAT, this training program will provide tailored feedback to individual raters and thus improve the effectiveness and efficiency of rater training in performance assessments. This work has been selected to receive a Provost’s Research Award for FY2022.